AI Network Automation by 7+ Use Cases & Case Studies in 2024
As network complexity and scale expand, the demand for efficient network management and automation increases. By 2025, artificial intelligence (AI)-driven automation solutions are projected to dominate over 95% of roles in supply chain management, with AI playing a critical role in nearly 40% of these positions.1
With the integration of AI, machine learning (ML), and advanced algorithms, AI-powered network automation brings unprecedented efficiency, reliability, and scalability to network operations.
This article summarizes the benefits, use cases, and technologies of both enterprise and telecom AI network automation journey by providing case studies.
The paradigm shift in AI network automation
AI network automation tools are essential to keep up with rapidly growing cyber threats. At the convergence of artificial intelligence, machine learning, and advanced algorithms lies AI-powered network automation, poised to revolutionize how networks are managed and optimized.
AI technologies such as machine learning, deep learning, and natural language processing have redefined how network security is approached. These AI tools can analyze vast amounts of data, identify patterns, detect anomalies, and make intelligent decisions without human intervention. By leveraging AI, organizations can achieve automated network security that is proactive, adaptive, and highly effective.
Benefits of AI-based network automation
Figure 1. Summarizes how much value AI network automation adds to businesses
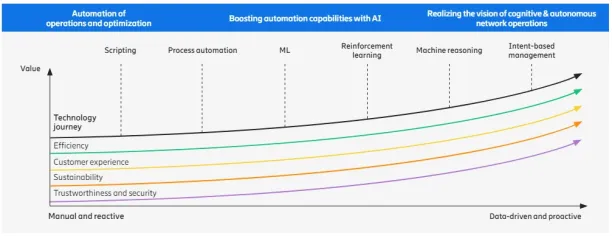
Source: Ericsson2
1. Enhanced efficiency and streamlined operations
Artificial intelligence-powered network automation streamlines operations by reducing manual configuration tasks, leading to more efficient resource utilization and manpower. By automating repetitive processes and workflows, organizations can achieve higher levels of productivity and operational excellence.
Case study
- AI network automation tools enhance efficiency and streamline operations by leveraging AI optimization software and services, as evidenced by Ericsson RAN customers-Far EasTone, Swisscom, and TDC NET-topping global benchmark rankings in 2021.3 They consistently outperform competitors, reaching a winning rate exceeding 90 percent when coupled with performance partnership network optimization services.
- Red Hat Ansible Automation Platform has significantly enhanced efficiency and streamlined ProRail operations by automating software installation and IT network management tasks. ProRail can now complete these tasks faster and more efficiently, as exemplified by the automation of changes in 3,000 switches that previously took days, now completed within several hours, saving valuable time and resources for the railway manager.
2. Improved network reliability
Proactive detection and prevention of network issues through AI-powered automation enhance network reliability and minimize downtime. Machine learning algorithms analyze historical network data to predict potential issues, allowing for preventive maintenance and minimizing the impact of failures.
Case study
- AI network automation tool significantly improves network reliability at the University of Oxford by enhancing network visibility through Juniper Mist AI and Wi-Fi Assurance. This technology enables quick troubleshooting, predicts potential issues, and ensures a predictable, reliable Wi-Fi experience for students and staff, even in historic buildings with unique challenges.4
3. Enhanced scalability and adaptive networks
AI-driven automation enables networks to seamlessly adapt to changing demands and traffic patterns, facilitating scalability and flexibility in infrastructure. This adaptability is crucial for accommodating the growth of IoT devices and data traffic, ensuring optimal performance under varying conditions.
Case study
- Ericsson’s AI tool assists in adapting to changing demands and traffic patterns by enabling scalability and flexibility in infrastructure, as demonstrated by Ooredoo Qatar’s successful management of over 44 million daily minutes of phone calls and seamless connectivity for 1.4 million visitors during a major sporting event in 2022.5
4. Faster response to anomalies and real-time security
Real-time anomaly detection and response capabilities of AI-powered automation enable networks to quickly detect and mitigate potential threats. This fast response to anomalies improves security posture and minimizes the potential damage caused by security breaches.
Case study
- Cisco’s AI Network Analytics, integrated into DNA Center, leverages machine learning to detect and respond to potential threats by identifying unusual patterns indicative of security risks or operational challenges, allowing for swift remedial measures and enhanced network management capabilities, as demonstrated by REWE Group’s streamlined network operations and reduced issue resolution time.6
5. Cost reduction by optimized resource allocation
Automation through AI-powered tools minimizes labor expenses and optimizes resource usage, resulting in significant cost savings for organizations. By reducing manual intervention and optimizing workflows, artificial intelligence driven network automation enhances operational efficiency and reduces operational costs.
Case study
- AI network automation tools optimize resource allocation and reduce costs by providing actionable insights and data-driven decision-making capabilities, as evidenced by the City of Philadelphia’s CityNet project. Leveraging Juniper’s solutions, such as the MX Series routers and Mist AI-driven automation, the city achieved improved network user experiences, enhanced security, and streamlined operations, empowering IT teams to focus on strategic value delivery while ensuring a reliable, agile network foundation.7
- Ericsson claims to achieve a 60% reduction in network performance issues for its customers through the use of Intelligent RAN automation.8
- AI network automation tool significantly reduces costs by optimizing resource allocation, as evidenced by the results achieved by UiPath’s RPA solution. With up to 3,700 tasks processed per day and 26 FTE/year saved on a single automated process, organizations can streamline operations, save time and resources, and focus on core business processes while ensuring high cybersecurity standards and system reliability.
AI network security automation technologies
Figure 2. Show how intent driven and incident driven operations change performance
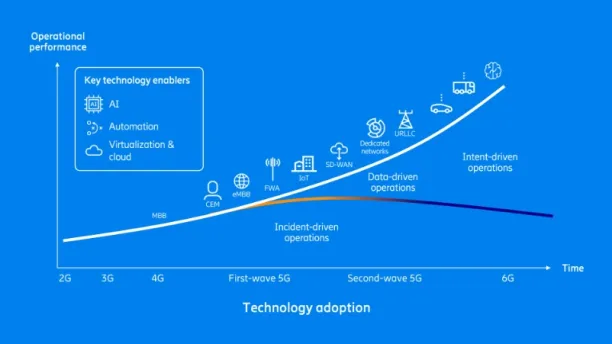
Source: Ericsson9
1. Machine learning algorithms
Machine learning algorithms are at the core of AI-driven network automation. ML algorithms analyze vast amounts of network data, detect patterns, and learn from historical network behavior. By leveraging machine learning, organizations can predict network issues, automate routine tasks, and make data-driven decisions for network optimization.10
Figure 3. Summarizes machine learning and deep learning algorithms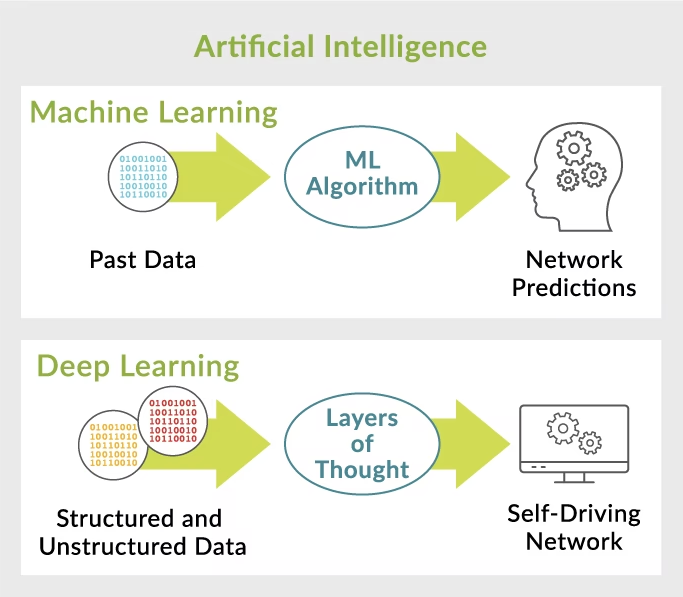
Source: Juniper11
1.1. Deep learning
Deep learning networks, a subset of machine learning, excel in handling complex and unstructured data. These networks can be trained to recognize anomalies, detect security threats, and optimize network configurations. Deep learning algorithms are particularly effective in real-time decision-making and automating complex network operations.
1.2. Natural language processing (NLP)
Natural language processing (NLP) is another powerful AI tool that can streamline network automation workflows. NLP algorithms can interpret human language, enabling network engineers to interact with automation systems using natural language commands. This simplifies tasks such as troubleshooting, provisioning, and configuration management, reducing the need for manual intervention.
4. Predictive analytics
Predictive analytics tools use AI algorithms to forecast future network performance, capacity requirements, and potential issues. By analyzing historical data and trends, these tools can proactively identify network bottlenecks, predict equipment failures, and optimize resource allocation. Predictive analytics empower organizations to take preemptive measures and prevent network disruptions.12
5. Robotic process automation (RPA)
RPA tools automate repetitive and rule-based tasks within network operations. By mimicking human actions, RPA bots can perform tasks like device configuration, software updates, and network monitoring with speed and accuracy. RPA enhances workflow efficiency, frees up valuable human resources, and ensures consistent execution of network tasks.13
Case study
- Network intelligent automation tool has significantly aided Heilbrigðisstofnun Norðurlands (HSN) in overcoming its business challenges and achieving transformative results. By streamlining HR and financial operations with swift and error-free data entry, processing, and management, HSN has experienced higher employee productivity, improved patient experiences through consolidated and updated data, and substantial cost savings exceeding 10 million Icelandic krónur (ISK) annually, thanks to over 20 AI based automated processes and a more cost-efficient RPA licensing model.
6. Network simulation and emulation
AI-powered network simulation and emulation tools create virtual environments to test network configurations, deploy new services, and assess performance impact. AI-driven simulations enable testing and validation of automation processes in a controlled environment by creating virtual representations of physical network environments through technologies like network digital twins (NDT).
Emulation further refines this process by replicating real-world network behavior, allowing AI algorithms to learn and optimize automation workflows without impacting live networks, thereby enhancing network automation efficiency and reliability.
7. Intent-based networking (IBN) solutions
Intent-based networking (IBN) automation solutions utilize AI and machine learning to translate high-level business objectives into actionable network policies. These solutions automate network provisioning, optimize resource allocation, and enforce security policies based on intent. Intent based network automation tools improve network agility, responsiveness, and alignment with business goals.
Use cases of AI-powered network automation
AI network automation plays a pivotal role in revolutionizing network operations and optimizing performance. By harnessing the advanced capabilities of AI, machine learning, and advanced algorithms, these tools perform a range of key roles that are instrumental in enhancing network efficiency, reliability, security, and scalability.
1. Predictive maintenance (PdM)
Machine learning algorithms analyze historical network data to predict potential issues or failures. This proactive approach to maintenance enables organizations to address problems before they escalate, minimizing downtime and improving overall network reliability.14
2. Real time anomaly detection
AI continuously monitors network traffic patterns and behavior to detect anomalies and security threats in real time. This capability allows for faster incident response times, ensuring that network vulnerabilities are promptly addressed to prevent potential breaches.
4. Configuration management automation
AI plays a crucial role in network configuration management automation by analyzing network configurations, identifying optimal settings, and automating deployment processes, resulting in streamlined operations and reduced human errors. This technology enables organizations to efficiently manage complex network infrastructures and ensure consistent configuration across diverse network devices and environments.
5. Quality of service (QoS) optimization
AI algorithms prioritize and manage network traffic to guarantee that critical applications and services receive the necessary resources and bandwidth. This optimization of QoS leads to improved user experiences and seamless delivery of services across the network.
Further Reading
- Analysis of Top 5 Open Source Security Automation Tools in 2024
- Analysis of Top 7 Network Security Automation Tools in 2024
- Top 10 Network Security Audit Tools in 2024: Based on 4,000 Reviews
External Links
- 1. appinventiv. The Role of Artificial Intelligence in Supply Chain Management. Accessed: 08/May/2024.
- 2. Ericsson. Intelligent automation. Accessed: 08/May/2024.
- 3. Ericsson. The story behind an award winning network. Accessed: 08/May/2024.
- 4. Juniper. AI-driven Wi-Fi takes digital experience honours at University of Oxford. Accessed: 08/May/2024.
- 5. Ericsson. Building an outstanding network with Ooredoo Qatar. Accessed: 08/May/2024.
- 6. Oswal, A. Cisco AI Network Analytics: Making Networks Smarter and Simpler to Manage. Accessed: 08/May/2024.
- 7. Juniper. Philadelphia Advances Smart City Vision. Accessed: 08/May/2024.
- 8. Ericsson. Intelligent Automation. Accessed: 08/May/2024.
- 9. Ericsson. AI-powered data-driven operations. Accessed: 08/May/2024.
- 10. Ma, L., & Sun, B. (2020). Machine learning and AI in marketing–Connecting computing power to human insights. International Journal of Research in Marketing, 37(3), 481-504. Accessed: 08/May/2024.
- 11. Juniper. What is artificial intelligence for networking? Accessed: 08/May/2024.
- 12. Aljohani, A. (2023). Predictive analytics and machine learning for real-time supply chain risk mitigation and agility. Sustainability, 15(20), 15088. Accessed: 08/May/2024.
- 13. Infopulse. Robotic Process Automation (RPA) Solution for European Safety Leader Accessed: 08/May/2024.
- 14. Infopulse. Using AI-Enabled Predictive Maintenance to help Maximize Asset Value. Accessed: 08/May/2024.

Cem is the principal analyst at AIMultiple since 2017. AIMultiple informs hundreds of thousands of businesses (as per Similarweb) including 60% of Fortune 500 every month.
Cem's work has been cited by leading global publications including Business Insider, Forbes, Washington Post, global firms like Deloitte, HPE, NGOs like World Economic Forum and supranational organizations like European Commission. You can see more reputable companies and media that referenced AIMultiple.
Throughout his career, Cem served as a tech consultant, tech buyer and tech entrepreneur. He advised enterprises on their technology decisions at McKinsey & Company and Altman Solon for more than a decade. He also published a McKinsey report on digitalization.
He led technology strategy and procurement of a telco while reporting to the CEO. He has also led commercial growth of deep tech company Hypatos that reached a 7 digit annual recurring revenue and a 9 digit valuation from 0 within 2 years. Cem's work in Hypatos was covered by leading technology publications like TechCrunch and Business Insider.
Cem regularly speaks at international technology conferences. He graduated from Bogazici University as a computer engineer and holds an MBA from Columbia Business School.
Sources:
AIMultiple.com Traffic Analytics, Ranking & Audience, Similarweb.
Why Microsoft, IBM, and Google Are Ramping up Efforts on AI Ethics, Business Insider.
Microsoft invests $1 billion in OpenAI to pursue artificial intelligence that’s smarter than we are, Washington Post.
Data management barriers to AI success, Deloitte.
Empowering AI Leadership: AI C-Suite Toolkit, World Economic Forum.
Science, Research and Innovation Performance of the EU, European Commission.
Public-sector digitization: The trillion-dollar challenge, McKinsey & Company.
Hypatos gets $11.8M for a deep learning approach to document processing, TechCrunch.
We got an exclusive look at the pitch deck AI startup Hypatos used to raise $11 million, Business Insider.
To stay up-to-date on B2B tech & accelerate your enterprise:
Follow on
Comments
Your email address will not be published. All fields are required.