Document Annotation: In-depth Guide & Use Cases in 2024
Documentation is an integral part of any business. Whether it’s in healthcare, technology, or the food sector, accurate documentation is vital to day-to-day and long-term operations.
Analyzing documents is a tedious and error-prone task. If done manually, it can lead to errors which ultimately transform into inefficiencies in the business. Document annotation can help resolve this problem.
This article explores what document annotation is, what are the types, use cases, and best practices to help business leaders ensure maximum return on their data annotation investments.
What is document annotation?
Document annotation is a type of data annotation which makes it easier to extract and learn information in documents without the need to manually read them. The process of document annotation includes identifying fields and values from a document and extracting information of value through criteria.
For example, through document annotation, information from this hotel bill can be, analyzed, stored, and easily extracted without going through the whole archive.
How does it work?
Document annotation involves labeling values and information in documents to train an ML model, which feeds this data into an AI-enabled document processing system. This system can organize, process, and present this data as the user requires (see Figure 1).
Some data is rejected and sent to be reviewed by a human annotator, who then updates the training model for similar scenarios in the future.
Figure 1. A simplified document annotation process
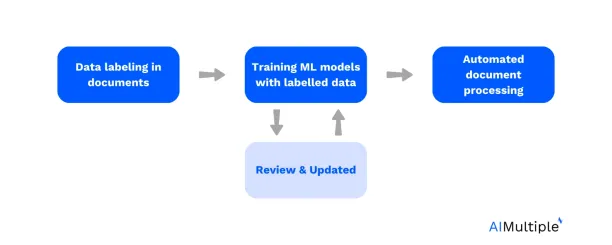
Watch how Microsoft leverages document annotation to provide automated document processing solutions:
What are the types of document annotation and use cases?
This section explains the types of document annotation and some real-world use cases of those types:
Named entity annotation
This type of data annotation is done by adding labels to specified words and phrases through named entity recognition (NER) technology. NER is used when the machine learning model needs to learn the subject matter of the written text.
Use cases:
- In customer service automation, customer requests can be directed to the relevant channel. Through the AI model, certain phrases can be linked directly to relevant channels.
- In human resources, named entity recognition document annotation can train ML models to identify information from resumes matching the job requirements.
- In the healthcare sector, NER document annotation can be used to train ML models to accurately analyze patient records and medical reports.
Sentiment annotation
This type of annotation refers to annotating the sentimental meaning behind the text. Sentiment annotation can help train the ML model to understand if the sentiment or emotion behind the phrase is negative, positive, or neutral.
Use cases:
- In digital or social medial marketing, sentiment annotation can be used to understand the meaning behind customer comments to get a better idea of the brand image.
- In human resources, sentiment annotation can be used to analyze a large number of employee satisfaction questionnaires.
To learn more about sentiment analysis, check out this quick read.
Semantic document annotation
This type of annotation involves labeling jargon and vague phrases. One common use case involves virtual assistants and chatbots to better understand customer queries with jargon.
What are some best practices for document annotation?
Here are some best practices that can help guide a document annotation project:
Annotate everything
Doing a thorough job is important in document annotation. A machine learning model learns from negative examples, the annotated documents, and the not annotated ones as well. For example, from 10 documents, if only the first 5 are annotated, and the other 5 are not, the machine learning model will learn to ignore the data that was present in the last 5 documents.
Consistency is key
Sometimes correctness is not as important as consistency in document annotation. For example, while annotating a car registration document, there can be variations in the name, like Civic and Civic XR. It is not important to know which name is correct for labeling rather than just picking one and continuing with the labeling.
Involve experienced annotators
Some large datasets require special expertise; therefore, it is beneficial to involve experienced annotators in the process. Experienced annotators can review the annotation and provide feedback.
You can also check our lists of data annotation tools and services:
Further reading
- Data annotation
- Video annotation
- Video annotation tools
- Medical data annotation
- Computer vision in healthcare
If you have any questions about document annotation, do not hesitate to contact us:

Comments
Your email address will not be published. All fields are required.